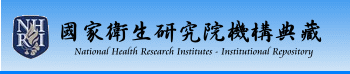 |
English
|
正體中文
|
简体中文
|
Items with full text/Total items : 12145/12927 (94%)
Visitors : 907449
Online Users : 966
|
|
|
Loading...
|
Please use this identifier to cite or link to this item:
http://ir.nhri.org.tw/handle/3990099045/14867
|
Title: | Deep-learning based breast cancer detection for cross-staining histopathology images |
Authors: | Huang, PW;Ouyang, H;Hsu, BY;Chang, YR;Lin, YC;Chen, YA;Hsieh, YH;Fu, CC;Li, CF;Lin, CH;Lin, YY;Chang, MDT;Pai, TW |
Contributors: | National Institute of Cancer Research |
Abstract: | Hematoxylin and eosin (H&E) staining is the gold standard for tissue characterization in routine pathological diagnoses. However, these visible light dyes do not exclusively label the nuclei and cytoplasm, making clear-cut segmentation of staining signals challenging. Currently, fluorescent staining technology is much more common in clinical research for analyzing tissue morphology and protein distribution owing to its advantages of channel independence, multiplex labeling, and the possibility of enabling 3D tissue labeling. Although both H&E and fluorescent dyes can stain the nucleus and cytoplasm for representative tissue morphology, color variation between these two staining technologies makes cross-analysis difficult, especially with computer-assisted artificial intelligence (AI) algorithms. In this study, we applied color normalization and nucleus extraction methods to overcome the variation between staining technologies. We also developed an available workflow for using an H&E-stained segmentation AI model in the analysis of fluorescent nucleic acid staining images in breast cancer tumor recognition, resulting in 89.6% and 80.5% accuracy in recognizing specific tumor features in H&E− and fluorescent-stained pathological images, respectively. The results show that the cross-staining inference maintained the same precision level as the proposed workflow, providing an opportunity for an expansion of the application of current pathology AI models. |
Date: | 2023-02 |
Relation: | Heliyon. 2023 Feb;9(2):Article number e13171. |
Link to: | http://dx.doi.org/10.1016/j.heliyon.2023.e13171 |
JIF/Ranking 2023: | http://gateway.webofknowledge.com/gateway/Gateway.cgi?GWVersion=2&SrcAuth=NHRI&SrcApp=NHRI_IR&KeyISSN=2405-8440&DestApp=IC2JCR |
Cited Times(WOS): | https://www.webofscience.com/wos/woscc/full-record/WOS:000969455900001 |
Cited Times(Scopus): | https://www.scopus.com/inward/record.url?partnerID=HzOxMe3b&scp=85147371383 |
Appears in Collections: | [其他] 期刊論文
|
Files in This Item:
File |
Description |
Size | Format | |
SCP85147371383.pdf | | 8444Kb | Adobe PDF | 148 | View/Open |
|
All items in NHRI are protected by copyright, with all rights reserved.
|